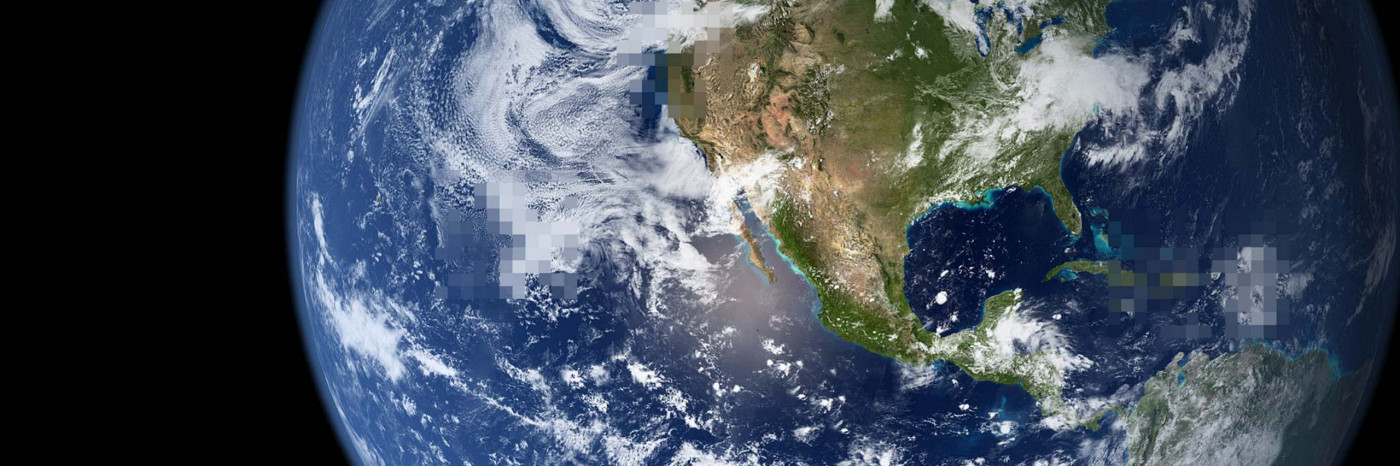
HIDA DATATHON for Grand Challenges on Climate Change
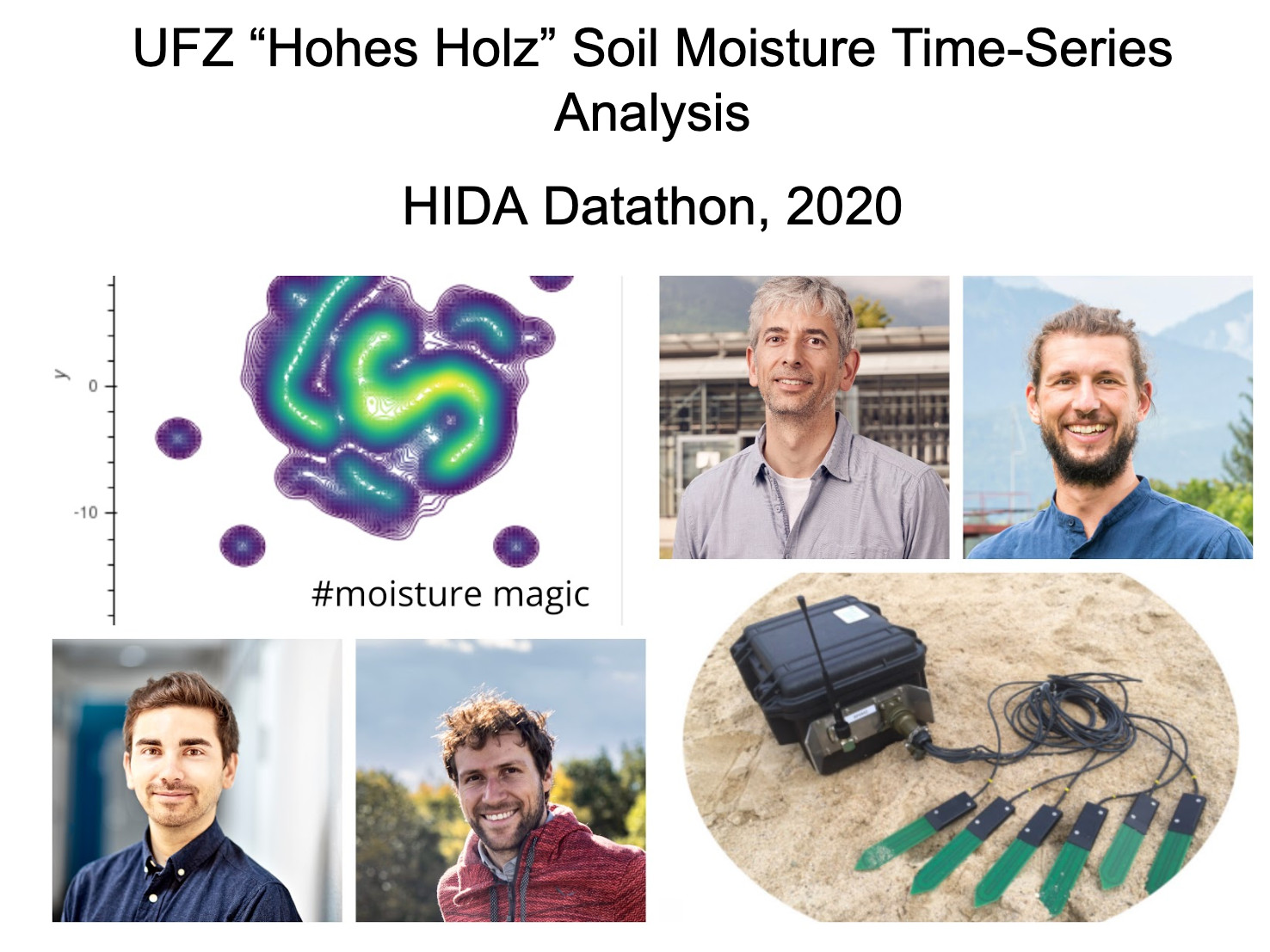
At the HIDA Datathon on 5-6. November 2020, scientists from the Helmholtz Association collected five current problems in the field of environmental science, the solution of which was promising for the application of methods in the field of "data science". Christian Werner, Maximilian Graf and Julius Polz took part in the challenge "Spot the mistake in ~ 50 million data points, cleverly" and won. The challenge was initiated by the UFZ in Leipzig. It was about the SoilNet soil moisture and temperature data from the TERENO "Hohes Holz" station, which has been measured for several years with the sensors developed at FZ Jülich. These sensors are dependent on a manual data quality control. The aim of the challenge was to automate this process, if possible without using the manual "quality flags" already collected. Accordingly, “unsupervised machine learning” is preferred over the current supervised algorithms, which have to know the “truth” for the learning process. The submitted solution to the problem consisted of two key components. First, the conversion of the partly unorganized data into a coherent time series format in order to generally enable machine learning. And secondly, the application of Uniform Manifold Approximation and Projection (UMAP) and subsequent "clustering" of the data in different categories. With this approach, all requirements of a solution, including a robust validation of the method, could be met within two days. The efficient and effective combination of different expert knowledge made the following end-to-end solution possible which was presented during the Datathon in this video. This solution is a first step and offers a variety of optimization potential. The approach is to be pursued in the future together with the UFZ, as it is suitable for many applications at the IMK-IFU and the University of Augsburg. The title "Supervised and unsupervised machine-learning for automated quality control of environmental sensor data" can be regarded as a project that is largely detached from data and which was also submitted as a contribution to this year's EGU conference in the session "Machine learning for earth system modeling".